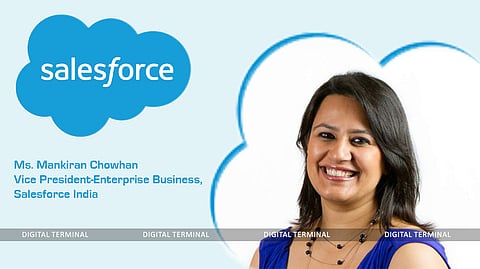
Authored by Mankiran Chowhan, Vice President - Enterprise Business, Salesforce India
This is a seminal moment for AI as advancements in generative capabilities captivate imaginations and drive business adoption. These models have the potential to change every company and deliver new levels of success for their customers. For the workforce, they can remove certain repetitive tasks and lower the usage barrier, creating new opportunities for those who don’t have specific skills to succeed in new spheres.
AI is driving long term organization-wide efficiency and transformation. Marketers, for example, are using AI to identify audience segments and auto-generate landing page images and copy. In sales, teams can easily compose emails, scheduling meetings, and prepare for the next interaction. In customer service, agents can generate knowledge articles from past case notes, auto-generate agent chat replies to increase customer satisfaction through personalized and expedited service.
Generative AI will transform the way we work, yet it is not without risks. It gets a lot of things right but many things wrong. Company leaders want to embrace generative AI but recognize the need to balance value and risk. In fact, a recent survey of more than 500 senior IT leaders revealed a majority (67%) are prioritizing generative AI for their business within the next 18 months, with one-third (33%) naming it as a top priority.
Developing AI Inclusively and Intentionally
Organizations need a clear and actionable framework for how to use generative AI and to align their generative AI goals with their business needs and values. It is critical that businesses consider trusted AI principles and embed ethical guardrails and guidance across products to help employees, partners and customers innovate responsibly — and catch potential problems before they happen.
Companies can provide trusted, open, real-time generative AI that is enterprise ready by bringing together AI, data, analytics, and automation.
To help guide the responsible and ethical development and use of these technologies, companies should lean on five guidelines.
Accuracy and trustworthy: Data is fuel for AI — without high-quality, trusted data, it becomes ‘garbage in, garbage out.’ It’s crucial its recommendations are reliable, that customers are enabled to train models on their own data and validate these responses. This can be done by citing sources, explainability of why the AI gave the responses it did and ensuring there’s a human in the loop when appropriate rather than relying entirely on automation.
Safety: Every effort to mitigate harmful outputs by conducting bias, explainability, and robustness assessments should always be a priority in AI. Organizations must protect the privacy of any personally identifying information (PII) present in the data used for training to prevent potential harm. Security assessments can help organizations identify vulnerabilities that may be exploited by bad actors.
Honesty: When collecting data to train and evaluate models, respect data provenance and ensure there is consent to use that data. When training models, using tags, for instance, teams can instruct their system to identify and not use training data including personal information in its output. We must also be transparent that an AI has created content when it is autonomously delivered. For example, a chatbot response to a consumer could include use of watermarks.
Empowerment: AI should play a supporting role to the human. By keeping a human in the loop approach when developing and using generative AI technologies, businesses can validate and test automated workflows with human oversight before unleashing fully autonomous systems and help build trust and confidence in the technology among stakeholders and customers.
Sustainability: Responsible AI also means sustainable AI. Language models are described as “large” based on the number of values or parameters it uses. When considering AI models, larger doesn’t always mean better. The bigger the model, the more energy and water is needed to run data centers powering them. As we strive to create more accurate models, we should develop right-sized models where possible to reduce our carbon footprint and water use.
The need for a Multi-Stakeholder Approach
It’s still early days of this transformative technology but learning and partnering with others will unlock the positive potential of AI. When it comes to regulation, no one company or organization has all of the answers. The real challenge for lawmakers is going to be dealing with the pace of change.
Adopting a multi-stakeholder approach across public and private sector, and civil society is crucial to identifying potential risk and sharing solutions to ensure these technologies are developed and used inclusively and with intention. Enterprises have the responsibility to ensure that they’re using this technology ethically and mitigating potential harm. Having guidelines and guardrails in place will help companies ensure that the tools they deploy are accurate, safe and trusted.